In a world where rural areas face increasing challenges and opportunities, it is essential to gain a deeper understanding of the dynamics that shape these regions. Nordregio is a part of GRANULAR – an EU project aimed at informing rural policies, by providing evidence at a more granular level to inform and improve policies.
One key dimension of rural development is the evolution of housing markets in rural areas. In remote regions in transition, like Norrbotten and Västerbotten in the northernmost territory of Sweden, where significant structural transformations are taking place due to ongoing green re-industrialization processes, understanding housing trends becomes crucial. These areas are anticipated to attract new populations and experience substantial changes in the housing market in the coming years.
Web scraping to understand rural housing development trends
To explore the housing markets in areas with limited publicly available statistics on housing markets, we tested an innovative approach to produce statistics: web scraping. Nordregio’s Senior researcher Carlos Tapia harnessed the power of the technique that mechanically gathers data from web pages. Web scraping involves using software to extract valuable data from online sources, including text, images, and tables.
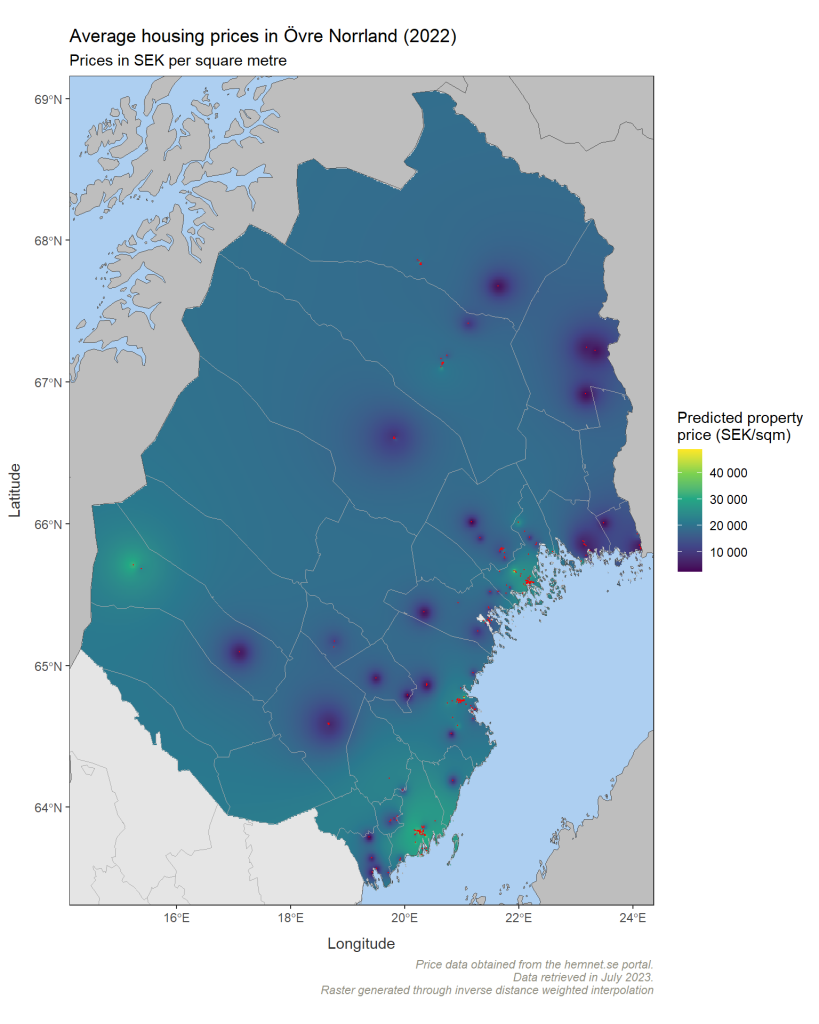
In GRANULAR, we use web scraping to study real-time property transactions using data from Hemnet, Sweden’s largest online housing marketplace. With around 200,000 homes listed on Hemnet annually, it provides a comprehensive sample of housing data in the country. These data help us understand local housing market performance in Sweden, especially in regions susceptible to external disruptions, and inform proactive responses to evolving challenges in these areas.
The Process
Data Collection: The initial step involved scraping data from Hemnet. The data collected includes property location, type, size, facilities, settlement dates, and price information. It’s a detailed snapshot of the housing market.
Data Cleanup: Although rich in information, scraped data normally require needed cleaning and structuring prior to utilisation, As part of this cleaning process, outliers were also removed to prepare data it for analysis.
Geocoding: Geocoding was essential to locate each property accurately. OpenStreetMap Nominatim was used for this purpose.
Interpolation: Creating housing price maps required interpolating the points. We employed inverse distance weighted interpolation and ordinary kriging to estimate prices for unsampled areas.
Potential impact
The implications of this approach go beyond the specifics of this demo. Data scraping may be a powerful tool for gaining insights into rural housing markets whenever official statistics are not available, enabling better policy decisions. In this case, we used it to investigate the impact of the Northvolt gigafactory on Skellefteå’s housing market. Here, a substantial increase of property prices could be observed in several parts of the inner city during the first half of 2023. This contrasts with the overall price drops observed during the same period in Region Västerbotten and Sweden as a whole and could be , potentially connected to the factory’s development.
Nordregio’s work in GRANULAR showcases how innovative data collection and analysis methods can provide a more detailed understanding of rural dynamics. For this, it is crucial to address issues related to data quality and availability, especially in regions with limited availability of statistical information at low territorial levels.
Read more
Via the button below, you can access the detailed report including methodology, process, chunks of code and interactive maps: “Collecting data to monitor housing markets in rural areas: a web scraping approach tested in the Övre Norrland region (Sweden)”.
The code is also available from the Carlos Tapia’s personal Github repository, here: https://carltap.github.io/housing-prices-demo/
In the quest to revitalise rural policies, GRANULAR’s work creates new datasets, tools and methods to better understand rural areas. By doing this, we hope to contribute to new insights into the unique characteristics, dynamics, and drivers of change in rural areas.